SPIN: Structure-Preserving Inner Offset Network for Scene Text Recognition
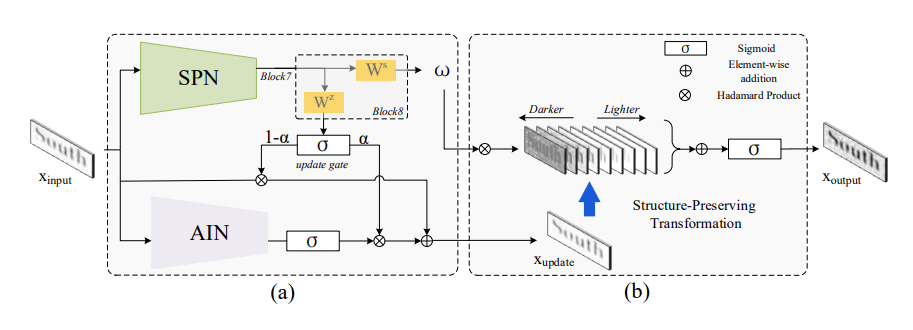
Abstract
Arbitrary text appearance poses a great challenge in scene text recognition tasks. Existing works mostly handle with the problem in consideration of the shape distortion, including perspective distortions, line curvature or other style variations. Therefore, methods based on spatial transformers are extensively studied. However, chromatic difficulties in complex scenes have not been paid much attention on. In this work, we introduce a new learnable geometric-unrelated module, the Structure-Preserving Inner Offset Network (SPIN), which allows the color manipulation of source data within the network. This differentiable module can be inserted before any recognition architecture to ease the downstream tasks, giving neural networks the ability to actively transform input intensity rather than the existing spatial rectification. It can also serve as a complementary module to known spatial transformations and work in both independent and collaborative ways with them. Extensive experiments show that the use of SPIN results in a significant improvement on multiple text recognition benchmarks compared to the state-of-the-arts. [Paper]Highlights Contributions
❃ To the best of our knowledge, it is the first work to mainly handle with the chromatic distortions in STR tasks, rather than the extensively discussed spatial ones. We also introduce the novel concept of inner and outer offsets and propose a novel module SPIN to rectify the images with chromatic transformation.
❃ The proposed SPIN can be easily integrated into deep neural networks and trained in an end-to-end way without additional annotations and extra losses. Unlike the typical spatial transformation based on STN, which is tied to tedious initialization schemes, the SPIN requires no need of sophisticated initialization, which enables it to be a more flexible module.
❃ The proposed SPIN achieves impressive effectiveness to recognize regular and irregular scene text recognition. Furthermore, the combination of chromatic and geometric transformations has been experimentally proved to be practicable and to outperform existing techniques on multiple benchmarks.
Recommended Citations
If you find our work is helpful to your research, please feel free to cite us:@inproceedings{DBLP:conf/aaai/0003XCPNWZ21, author = {Chengwei Zhang and Yunlu Xu and Zhanzhan Cheng and Shiliang Pu and Yi Niu and Fei Wu and Futai Zou}, title = {{SPIN:} Structure-Preserving Inner Offset Network for Scene Text Recognition}, booktitle = {AAAI}, pages = {3305--3314}, year = {2021}, }