Dynamic Low-Resolution Distillation for Cost-Efficient End-to-End Text Spotting
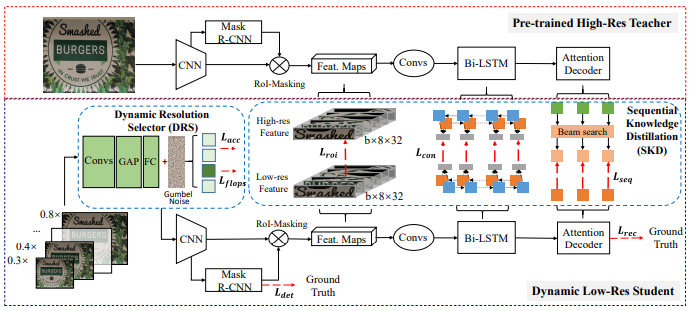
Abstract
End-to-end text spotting has attached great attention recently due to its benefits on global optimization and high maintainability for real applications. However, the input scale has always been a tough trade-off since recognizing a small text instance usually requires enlarging the whole image, which brings high computational costs. In this paper, to address this problem, we propose a novel cost-efficient Dynamic Low-resolution Distillation (DLD) text spotting framework, which aims to infer images in different small but recognizable resolutions and achieve a better balance between accuracy and efficiency. Concretely, we adopt a resolution selector to dynamically decide the input resolutions for different images, which is constraint by both inference accuracy and computational cost. Another sequential knowledge distillation strategy is conducted on the text recognition branch, making the low-res input obtains comparable performance to a high-res image. The proposed method can be optimized end-to-end and adopted in any current text spotting framework to improve the practicability. Extensive experiments on several text spotting benchmarks show that the proposed method vastly improves the usability of low-res models. [Paper]Highlights Contributions
❃ We first study the input resolution problem on end-to-end text spotting tasks and propose a Dynamic Low-resolution Distillation text spotting framework that can effectively enhance the performance and reduce the computational cost.
❃ We propose a sequential KD strategy with a dynamic resolution selector that allows the model to choose a small but recognizable input scale.
❃ Extensive experiments and ablation studies demonstrate the effectiveness of our method.
Recommended Citations
If you find our work is helpful to your research, please feel free to cite us:@inproceedings{ying@eccv2022, author = {Ying Chen and Liang Qiao and Zhanzhan Cheng and Shiliang Pu and Yi Niu and Xi Li }, title = {Dynamic Low-Resolution Distillation for Cost-Efficient End-to-End Text Spotting}, booktitle = {ECCV}, year = {2022}, }