LGPMA: Complicated Table Structure Recognition with Local and Global Pyramid Mask Alignment
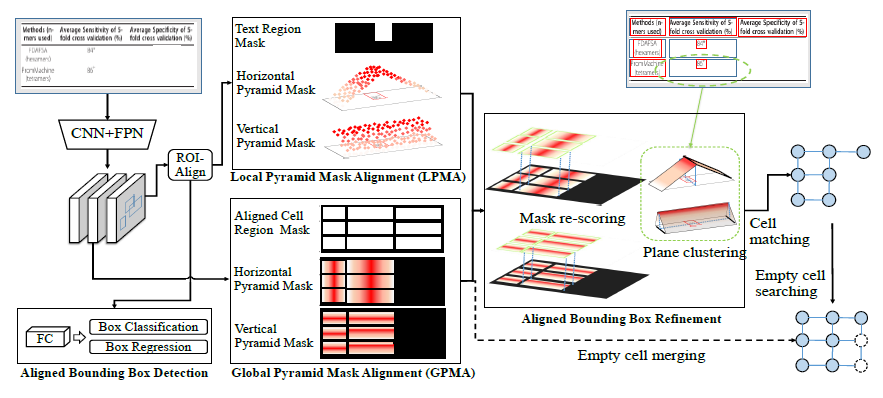
Abstract
Table structure recognition is a challenging task due to the various structures and complicated cell spanning relations. Previous methods handled the problem starting from elements in diferent granularities (rows/columns, text regions), which somehow fell into the issues like lossy heuristic rules or neglect of empty cell division. Based on table structure characteristics, we find that obtaining the aligned bounding boxes of text region can effectively maintain the entire relevant range of different cells. However, the aligned bounding boxes are hard to be accurately predicted due to the visual ambiguities. In this paper, we aim to obtain more reliable aligned bounding boxes by fully utilizing the visual information from both text regions in proposed local features and cell relations in global features. Specifically, we propose the framework of Local and Global Pyramid Mask Alignment (LGPMA), which adopts the soft pyramid mask learning mechanism in both the local and global feature maps. It allows the predicted boundaries of bounding boxes to break through the limitation of original proposals. A pyramid mask re-scoring module is then integrated to compromise the local and global information and refine the predicted boundaries. Finally, we propose a robust table structure recovery pipeline to obtain the final structure, in which we also effectively solve the problems of empty cells locating and division. Experimental results show that the proposed method achieves competitive and even new state-of-the-art performance on several public benchmarks. [Paper]Highlights Contributions
❃ We propose a novel framework called LGPMA Network that compromises the visual features from both local and global perspectives. The model makes full use of the information from the local and global features through a proposed mask re-scoring strategy, which can obtain more reliable aligned cell regions.
❃ We introduce a uniform table structure recovering pipeline, including cell matching, empty cell searching, and empty cell merging. Both non-empty cells and empty cells can be located and split effcaciously.
❃ Extensive experiments show that our method achieves competitive and even state-of-the-art results on several popular benchmarks.
This paper obtains the reward of ICDAR 2021 best industry paper!
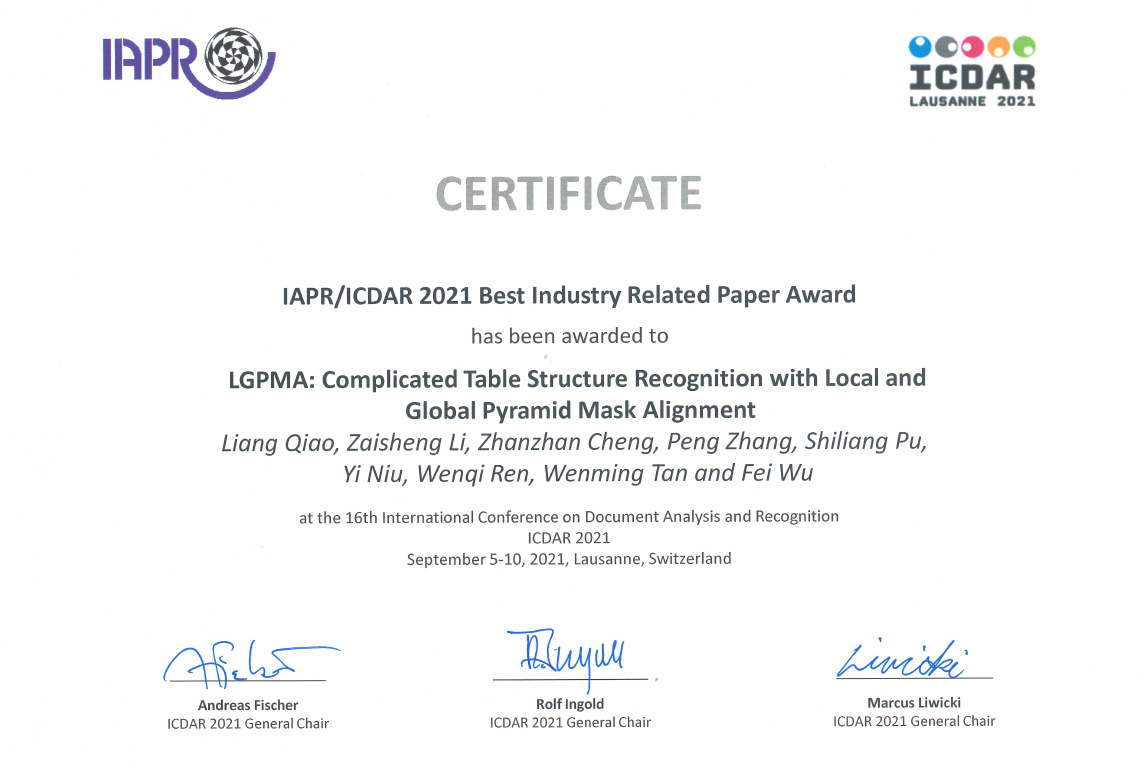
Recommended Citations
If you find our work is helpful to your research, please feel free to cite us:@inproceedings{qiao2021lgpma, author = {Liang Qiao and Zaisheng Li and Zhanzhan Cheng and Peng Zhang and Shiliang Pu and Yi Niu and Wenqi Ren and Wenming Tan and Fei Wu}, title = {{LGPMA:} Complicated Table Structure Recognition with Local and Global Pyramid Mask Alignment}, booktitle = {ICDAR}, volume = {12821}, pages = {99--114}, year = {2021}, }