Text Recognition in Real Scenarios with a Few Labeled Samples
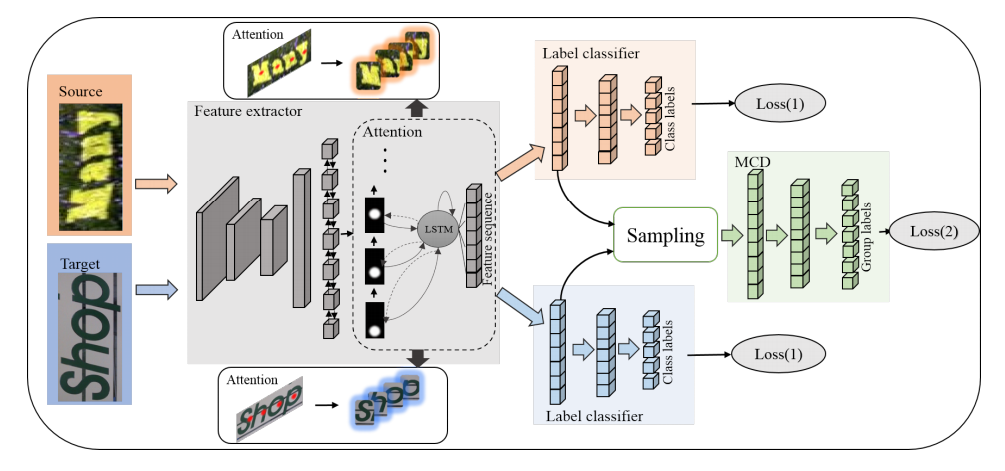
Abstract
Scene text recognition (STR) is still a hot research topic in computer vision field due to its various applications. Existing works mainly focus on learning a general model with a huge number of synthetic text images to recognize unconstrained scene texts, and have achieved substantial progress. However, these methods are not quite applicable in many real-world scenarios where 1) high recognition accuracy is required, while 2) labeled samples are lacked. To tackle this challenging problem, this paper proposes a few-shot adversarial sequence domain adaptation (FASDA) approach to build sequence adaptation between the synthetic source domain (with many synthetic labeled samples) and a specific target domain (with only some or a few real labeled samples). This is done by simultaneously learning each character's feature representation with an attention mechanism and establishing the corresponding character-level latent subspace with adversarial learning. Our approach can maximize the character-level confusion between the source domain and the target domain, thus achieves the sequence-level adaptation with even a small number of labeled samples in the target domain. Extensive experiments on various datasets show that our method significantly outperforms the finetuning scheme, and obtains comparable performance to the state-of-the-art STR methods. [Paper]Highlights Contributions
❃ We propose a few-shot adversarial sequence domain adaptation approach to achieve sequence-level domain confusion by integrating a well-designed attention mechanism with sequencelevel adversarial learning strategy into a framework.
❃ We implement the framework to fill the performance gap between general STR models and specific STR applications, and show that the framework can be trained end-to-end with much fewer sequence-level annotations.
❃ We conduct extensive experiments to show that our method significantly outperforms traditional learning-based schemes such as finetuning, and is competitive with the state-of-the-art STR methods.
Recommended Citations
If you find our work is helpful to your research, please feel free to cite us:@article{lin2020fasda, title={Text Recognition in Real Scenarios with a Few Labeled Samples}, author={Lin, Jinghuang and Cheng, Zhanzhan and Bai, Fan and Niu, Yi and Pu, Shipliang and Zhou, Shuigeng}, journal={arXiv preprint arXiv:2006.12209}, year={2020}, }